How Can Your AI-driven Business Benefit From Synthetic Data in 2024?
- AI/ML
- November 23, 2023
In this data and AI-driven age, whoever owns more data has much potential to do business impressively, make someone vouch for them, or play their malicious games. While sharing data with someone can seem okay for the personalized experience, it can also cause trouble on the other side due to privacy concerns.
- What if you could share data with anyone without worrying about breaching the privacy act?
- What if you can actually create a new revenue stream by selling your data to someone while ensuring the highest security and privacy standards?
- What if you can actually get relevant data that no one has and train your AI uniquely?
Yes, with the help of Synthetic Data, you can all make it possible!
Gartner estimates that almost all data to be used in training AI and analytics projects will be generated synthetically and it will overshadow the use of real data by 2030.
As per DataHorizzon Research, the market size of synthetic data generation is projected to touch $6.9 Billion by 2032, at a CAGR of 37.5%.
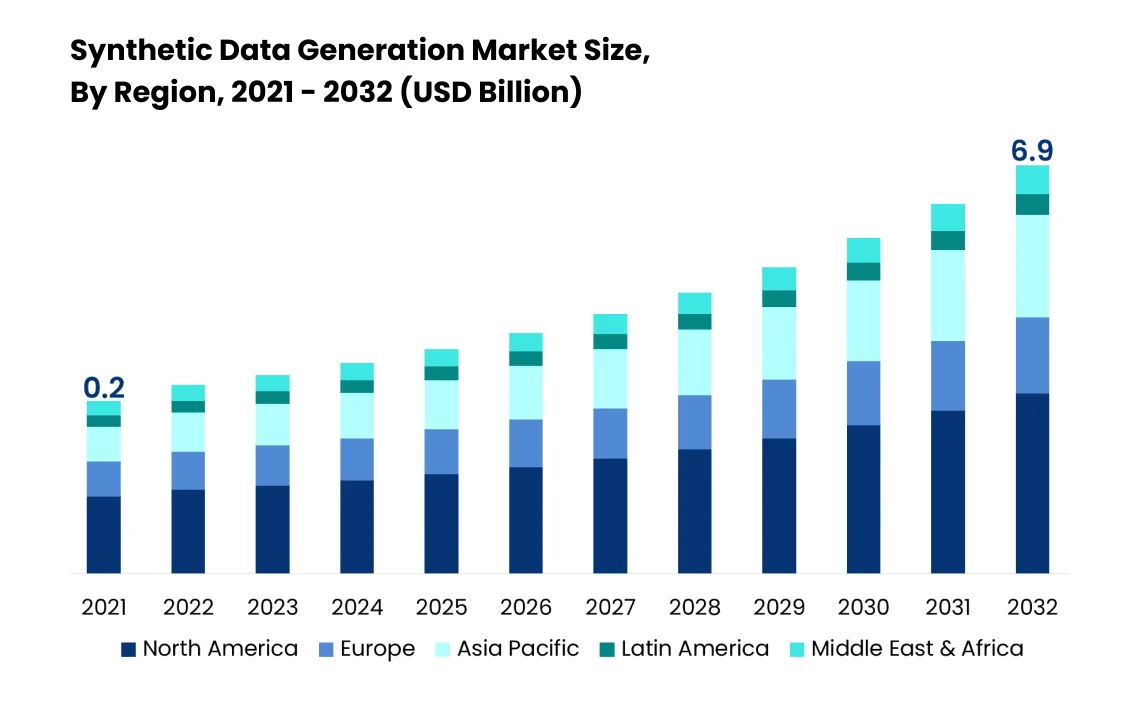
After seeing these numbers, we can say that this concept has gained the spotlight with the evolution of AI and has been playing a pivotal role across industries in various ways.
That’s quite impressive, right? In this blog, we have covered everything you need to know about synthetic models, from introduction to them, their use cases, and benefits to how to effectively generate synthetic data.
So, let’s dive into the ocean of synthetic data generation!
What is Synthetic Data?
Synthetic data is computer-generated, artificial data generated with the help of algorithms running on the input of real data or manually created data, which you want to populate. It is designed to improve AI models, protect sensitive data, and mitigate biases. To go more in-depth, to generate synthetic data, data experts use statistical methods, machine learning (GAN- Generative Adversarial Networks, a class of machine learning framework), or the combination of both.
The algorithms first analyze the training data and then reproduce data based on its statistical patterns to create many unique variations mimicking the same characteristics and predictive power as the original data. While real-world data is generated by real systems (such as medical tests or banking transactions), synthetic data is generated using mathematical models or algorithms.
Unlike real data that has many security clauses, synthetic data allows you to share it with anyone you want to without revealing any sensitive information of any particular.
Synthetic data can be generated in various forms like Text, Image, Video, and Audio, with many representation formats.
You can utilize synthetic data for various purposes, including training AI models, validating models, and testing new products and tools.
What Value does Synthetic Data create for your Business?
You may find this concept of synthetic data generation and modeling to be an emerging concept. But surely, in the later years, it will sound quite effective and will be adopted by many businesses dealing with data and AI as it offers the following three “S” benefits:
Security: Sensitive Information Protection
For example, when you’re sharing real data with someone who is training in AI/ML models, what’s the guarantee they will not misuse that data, which could have someone’s personal data? Thanks to synthetic data, you can avoid those security risks by generating relevant data, mimicking real one, and sharing those with third parties.
Speed: Quick Access to Data
You want to train your AI/ML models quickly to put them into action as soon as possible to start generating value for your business, but dealing with real-time data can be a tough nut to crack. As synthetic data deals with security and privacy parts, it removes that dependency and sets you free from cross-verifying cases to fall into the rule-breaking clauses (acting as roadblocks to achieving efficiency) and utilizes that time to strengthen your ML model.
Apart from that, businesses can also quickly train their ML models on datasets regardless of the size and length of datasets. It indirectly leads to achieving efficiency in training, testing, and deploying your AI solution in the marketplace.
Through this, data scientists and ML engineers working on your AI project get more confidence for the model development stages. With this confidence, they will also help you market your product quickly and generate business value faster.
Scale: A Bandwidth to Deal with Data and Problems at Scale
If you are getting better security and speed for your AI solution, then scaling of it will come with it as a complimentary gift. Technically speaking, access to security and speed offers better capability to analyze data at scale and thus solve more problems.
This benefit offered by synthetic data can sound more helpful for enterprises, depending only on current data generated through their AI-powered product, to scale their AI capabilities and solution accuracy and offer better user and customer experience.
What are the Benefits of using Synthetic Data Over Real Data?
Collecting real-life data from different sources and that too in different contexts can be a mind-busting process. For example, to train autonomous vehicles, you cannot rely on original data; it does require some simulations to run. For this, synthetic data comes into play. Apart from that, below are the reasons why synthetic data win, and hence, you should adopt that:
You Can Create Synthetic Data As Per Your Needs
As the synthetic data is generated through algorithms based on simulation, it has its ups and downsides. The upside is that you have control over what kind of data you want to generate in what quantity while controlling event frequency, object distribution, etc.
The downside is that there are chances for the synthetic data to miss edge cases, which are often created in real datasets through real-life interaction scenarios. However, the downside can be avoided by creating hybrid datasets, a combination of synthetic data with a sprinkle of some rare cases of real datasets. Apart from that, you can also manipulate synthetic datasets to test and train ML models more precisely.
Hence, it’s all up to you and your synthetic data modeling service provider to create a quality of synthetic data that’s best for your AI solution.
You Can Create Synthetic Data to be a Multispectral One
This kind of data is often helpful for automotive and auto-tech companies creating autonomous vehicles. The reason is the difficulty level in annoying the non-visible data.
Therefore, the biggest autonomous vehicle developing companies, like Alphabet’s Waymo, Amazon’s Zoox, NVIDIA, General Motors’ Cruise, and many others, are using generated synthetic LiDAR Data for easy data labeling and infrared or radar computer vision applications to unlock efficiency to train their self-driving car technology.
Synthetic data helps to label data to that level where human intelligence also sometimes lags in interpreting the imagery.
Get Over Biased Datasets
When there’s a requirement to get diverse data, sharing the same contextual values but with different characteristics, at that time, a Large Language Model (LLM) is suggested. This model often sounds effective in generating different variations of the synthetic data, which are as effective as real data versions, training your ML models.
This way, you can prepare your ML models in various conditions for various situations without letting them follow biases that their counterparts often follow.
Finding Specific Real Data Can Be Time-Intensive
We all can agree that finding real-life data for the conditions you require can be a time-consuming and tiresome process due to its rarity. Also, they are generated in very sufficient quantities. And, of course, you cannot rely on less data to train your AI model.
Hence, for the speed and quantity measures, you must opt for the generative synthetic data to train your AI for various scenarios and offer robust performance to your customers.
Cost-effective than Gathering Real Data
As the time is saved in generating synthetic data, it reduces the extra efforts involved in getting data at scale in hand. As its most effective benefit, synthetic data helps us mitigate privacy concerns by eliminating need for the data de-identification and privacy protection, thus, its associated costs.
Labeling real data can be a headache for us as we have our limitation to name each and every geographical and real-world property, while synthetic data is often generated with labels, saving extra time and cost associated with data labeling.
As it’s algorithmically generated, we have control over what type of data to start with; hence, eliminating data cleaning, harmonization, and organization needs post-data collection.
Lays off Security Worries and Regulatory Restrictions
We now know that synthetic data is extensively known for offering data generation by replicating all statistics properties of the real data while ignoring or not using properties, raising concerns for privacy regulations.
In real data used for AI/ML training purposes, it’s often difficult to sustain privacy while ensuring its usefulness. Either you can choose to protect the data privacy or ensure its usefulness by renouncing privacy. In short, to get one value, you have to lose another.
Thanks to the findings, with synthetic data, you can achieve both data privacy and usefulness. You can share your synthetic data with third parties for their research and simulation purposes and even as your monetization tool.
Achieve Better Data Consistency with Speed
Real data can come in a variety of forms, but when training your AI/ML solutions, uniformity and consistency are a must, which synthetic data helps to achieve. The uniformity in the synthetic datasets makes it more effective for the most accurate analysis and simulations.
Also, based on the given inputs for the statistical properties, these datasets are generated at speed with more accuracy.
Moreover, the simplicity of the synthetic data generation process helps to get quality and quantity of data in less time and effort without compromising on the data quality.
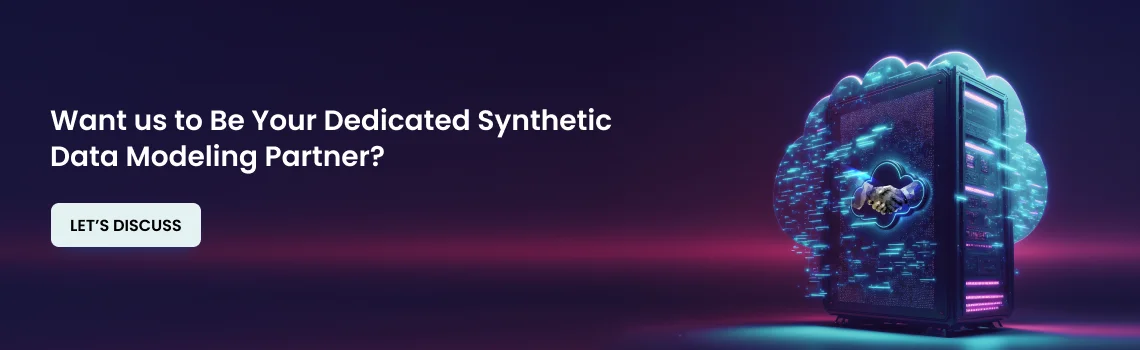
How to Generate Synthetic Data?
First, you have to figure out the exact type of synthetic data you want to generate. Then you have to consult a synthetic data specialist to finalize the technique for synthetic data based on the type of accuracy and format you want to have. Here are the popular techniques extensively used to generate synthetic datasets with accuracy:
Random Data Generation
Unlike other processes, random synthetic data generation is more of a general concept that doesn’t specify a particular distribution nor any specific pattern or predictability. It will help to create data randomly at scale; however, some patterns would be detectable and hard to foresee. Based on its quickness and scale, it’s often the best for applications such as simulations, cryptography, testing, and more.
Popular random generation methods used are Pseudorandom Number Generators (PRNGs), True Random Number Generators (TRNGs), Hardware Random Number Generators (HRNG), and more.
Drawing Data from a Distribution
It’s way similar to random data generation with a little bit of difference and the use of probability distribution functions.
However, it is the most popular technique used by data scientists and AI/ML experts, in which they draw out or just sample numbers at scale by generating data points following the characteristics of a defined probability distribution. As this method does not extract insights from real-world data, it creates loosely coupled datasets based on real-world data.
Generative Models
We are living in this age where Generative models and AI is becoming everyone’s favorite tool, and it’s the most advanced technique for synthetic data generation. It’s also known as unsupervised learning, which automatically discovers and learns insights and patterns that can further be used to generate new examples sharing the same statistics values as if it’s trained on real-world data.
When it comes to generative models there are two ways to use those:
Generative Adversarial Networks (GANs): In this, the training process is simulated with data coming from two networks: A Generative Network – that creates synthetic data instances and a Discriminator – that evaluates their authenticity. Through this process, the generator learns to produce more and more realistic data.
With each training iteration adding up, it generates data more accurately and to the extent that even discriminators find it difficult to differentiate between real and synthetic data. Moreover, GANs can be used to generate synthetic data of any format, including image, text, and more.
Large Language Models (LLMs): These though may seem like simpler ones, they are the most powerful algorithms to generate synthetic data. They provide the generation texts of any length based on training facilitated on data at scale.
Recurrent Neural Networks, like Long Short-Term Memory (LSTM) or Gradient-based Regularized Optimization (GRO) networks, and transformers like OpenAI’s GPT versions, Bidirectional Encoder Representations from Transformers (BERT), Text-To-Text Transfer Transformer (T5), etc., are used as language models, which can look into the past and the future both at the same time, to generate synthetic data without data recursion.
Data Augmentation
Generating synthetic data post-data augmentation is the most popular technique in data science and machine learning. It asks for augmenting real data and creating new data points by applying various transformations to such. It helps to scale the datasets, improve model generalization, and address class imbalance.
- Image Data Augmentation: Such media files can be augmented by applying techniques, including rotation, flipping, scaling, cropping, brightness adjustments, etc.
- Text Data Augmentation: Here, NLP is used with techniques like synonym replacement, paraphrasing, or adding random content to text data.
- Audio Data Augmentation: This can be done by applying pitch shifting, time stretching/compressing, adding background noise, or simply with frequency modulation.
- Tabular Data Augmentation: This structured data can be augmented by duplicating and modifying existing rows with random noise or applying statistical operations to numerical features.
- Time Series Data: This type of synthetic data is created by resampling, introducing random fluctuations, or applying Fourier transformation.
Variational Autoencoders (VAEs)
A Variational Autoencoder (VAE) is a type of deep neural system trained by objective function and is also quite popular amongst data scientists and AI/ML experts for generating synthetic data. Some experts also refer to VAE as a “black box”, an unsupervised machine learning method for synthetic data generation. In this, new data points are created similar to real data to be used for training purposes.
VAE consists of an Encoder Network that maps the input data into probabilistic distribution in the latent space, which ensures continuous and structured representation of the data. During the generation process, VAEs sample points from the latent space distribution. Then, the decoder network takes the sampled point and maps back to the data space to generate synthetic data.
How Is Synthetic Data Revolutionizing Different Industries?
Though having is quite a popular use case, which is to train AI/ML solutions, you can utilize synthetic data for various applications based on your industry type:
Healthcare
The healthcare industry is the most compliant industry (by HIPAA compliance), where they have to ensure patients’ data privacy (medical history, current health status, payment information, etc.) over any other.
However, one Accenture survey revealed that around 18% of healthcare employees get ready to sell patients’ confidential data to unauthorized parties for just $500 – $1000.
This concern creates a need to adopt AI in healthcare organizations in various ways to protect sensitive information.
Also, in the healthcare research and treatment procedures, HealthTech professionals would require data to continue their research. Due to privacy concerns and HIPAA compliance, it may become difficult to share patients’ data. Hence, synthetic data for healthcare organizations is needed.
Then, synthetic data becomes great for healthcare organizations for the following purposes:
- Creating and sharing de-identified datasets for research, analysis, and AI-powered healthcare software development
- Simulating patient data to help medical professionals practice procedures, diagnose diseases, and enhance their skills
- To develop personalized treatment plans for patients with rare or specific symptoms
- To get a boost on privacy-preserving research
- To run pharmaceutical simulations and get help in drug discovery
Finance
What if we share your personal details with any third party? Will you allow us? Of course not! Instead, file a lawsuit against us for violating your privacy rights. And to train your AI-powered fintech solution, you don’t want to go through extensive real data-gathering processes or fall into lawsuits. Therefore, synthetic data is a great help.
Among others, you can utilize synthetic data for the following use cases:
- To share data with other fintech research teams to collaborate with for the industry innovation
- Generating synthetic data through generative adverbial networks for fraud detection and prevention
- To support financial risk management applications
Cybersecurity
Every day, cyber attackers are finding new ways to hack into our network for their malicious gameplay. To stop them, you have to train your AI-powered network to learn every possible way to hack into it, and for that, attack drills are required.
Considering that, you can utilize synthetic data to conduct smart security bridge drills and boost the threat detection capabilities of the firewall.
Entertainment
The entertainment industry can also benefit from synthetic data generation to get more advanced content generation experience.
In reality, the Lord of the Rings: The Two Towers was the first cinematic battle that was designed with the use of advanced AI-powered visual effect software named Massive, which helps to rapidly populate the audience to be represented in a scene with distinct behaviors and actions.
Real Estate
In the real estate industry, synthetic data can be quite helpful to do the software-based interior planning of any property.
In one of our client projects, named – Passio.AI, we got an opportunity to work on its three different AI products, and one of them is Paint.AI. It allows users to virtually augment the color of their different walls of the room to perfectly plan the color-side interior.
When training its ML model, we faced a challenge in making it learn which object close to the wall is not a wall but a different entity, and when clicking on the wall, it should not paint that object as well.
As a solution, we took the help of synthetic data and Unity engine to create different variations of room images with different placements of room objects, along with sunlight and reflections, in the RGB image and Semantic Segmentation forms. With this image-based synthetic data, we’re able to achieve above 90% accuracy on the outputs of this AI product.
How can MindInventory Help you with Synthetic Data Modeling?
The demand for the data in this digital will always be on the rise. If all organizations and businesses open doors to their data, but in a synthetic way, there’s a very bright future to train your AI to the next extent. This will surely give birth to the synthetic data economy, which can definitely be used for a good cause without any violation of data privacy rights. Simply, the more access to data, the better intelligent AI systems can come into existence with faster time-to-market.
In this blog, you have gone through the kind of revolution synthetic data can bring to every conceivable industry. As it’s cutting-edge data science, the earlier you are to adopt it for your transformative AI project alongside fortune AI-based businesses, the more benefits you can reap from it and take your business to the top.
So, what are you waiting for? At MindInventory, we have a team of ingenious data scientists and a dedicated AI/ML development team who will be at your service and help you transform your vision into an intelligent digital solution. Contact us now to know the possibilities we can create for your Data Science and AI/ML project.
FAQs About Synthetic Data Modeling
If we see the bright side of AI becoming the mainstream function to be operated by human beings in this world, by seeing in the probable future, synthetic data does take a stand in shaping AI – we expect it to be. In this era, where many AI forecasters are believed to be running out of unique data, synthetic data can come as a ray of hope, a catalyst in building well-trained models, benefiting many research and development fields.
As the synthetic data sounds like a promising solution for your AI/ML development with its precision, it also comes with challenges of its own level. It may include creating data that accurately mirrors real-world complexity, ensuring models trained on synthetic data perform well with real data, the generated data does not reveal sensitive information, should be generalized well to different use cases, dealing with complex data structure, and ensuring that it does not inherit the bias of the original data.
When generating synthetic data, you have to consider magnifying potential biases that synthetic data can inherit, risks of re-identification and sensitive data exposure, not to use misapplication in contexts with stricter ethical regulations, not generating data without obtaining consent, all necessary accountability and transparency standards, ethical concerns in applications like deep-fake technology, not over-rely on synthetic data.